Unwrapping 2024: A look back at LLM-powered innovations from Inscribe
This year at Inscribe has been nothing short of transformative, marked by significant growth and innovation.
We embraced cutting-edge advancements in large language models (LLMs) from pioneers like Anthropic, which propelled our efforts to combat fraud and streamline workflows for risk teams.
A key milestone was the launch of AI Risk Agents, beginning with the AI Fraud Analyst — and there’s more to come. And this is just the beginning. As we look ahead, it’s easy to imagine a world where AI Agents are an integral part of every risk team in financial services. These AI-driven risk teams will streamline operations, reduce the need for manual input, and, most importantly, enable financial services companies to deliver a better, faster, and more trustworthy experience for their customers.
In previous years, we celebrated our top five risk-reducing features launched. However, this year’s achievements transcend features alone. Instead, we’re proud to highlight our LLM-powered innovations from 2024, showcasing our LLM advancements by the numbers:
9.5M LLM calls
This year, we recorded an impressive 9.5 million API calls to LLMs. These calls weren't just numbers; they drove meaningful actions, such as identifying suspicious patterns, enriching transaction data, and providing clearer insights for risk teams.
150% faster delivery
Inscribe reducedmodel training and deployment time by 150%, enabling faster delivery of tailored solutions to our customers. This is critical as it allows us to release updates that help our customers catch new types of fraud as they’re identified.
10+ LLM-enabled features
We launched over 10 new LLM-powered features, including a powerful, new fraud signal that checks bank statement summary sections for calculation errors or inconsistencies, triggering alerts for potential tampering or misrepresentation. A huge time-saver for teams manually reviewing bank statements today.
Three upgraded features
Inscribe upgraded three major features, including Transaction Enrichment, to use LLM technology, with more updates on the horizon. With Transaction Enrichment, Inscribe categorizes transactions so you can easily identify income, NSFs, withdrawals, self-transfers, loan repayments, and other transaction types in just seconds. We also provide merchant names to help you understand who your customers are already doing business with.
One AI Risk Agent built
While we mentioned it above, we had to mention it again! We introduced our first AI Risk Agent, the AI Fraud Analyst, which is helping risk teams automate manual fraud reviews so they can spend less time on in-depth investigations and more time converting trustworthy customers. Inscribe customers are reducing manual fraud reviews by 85%!
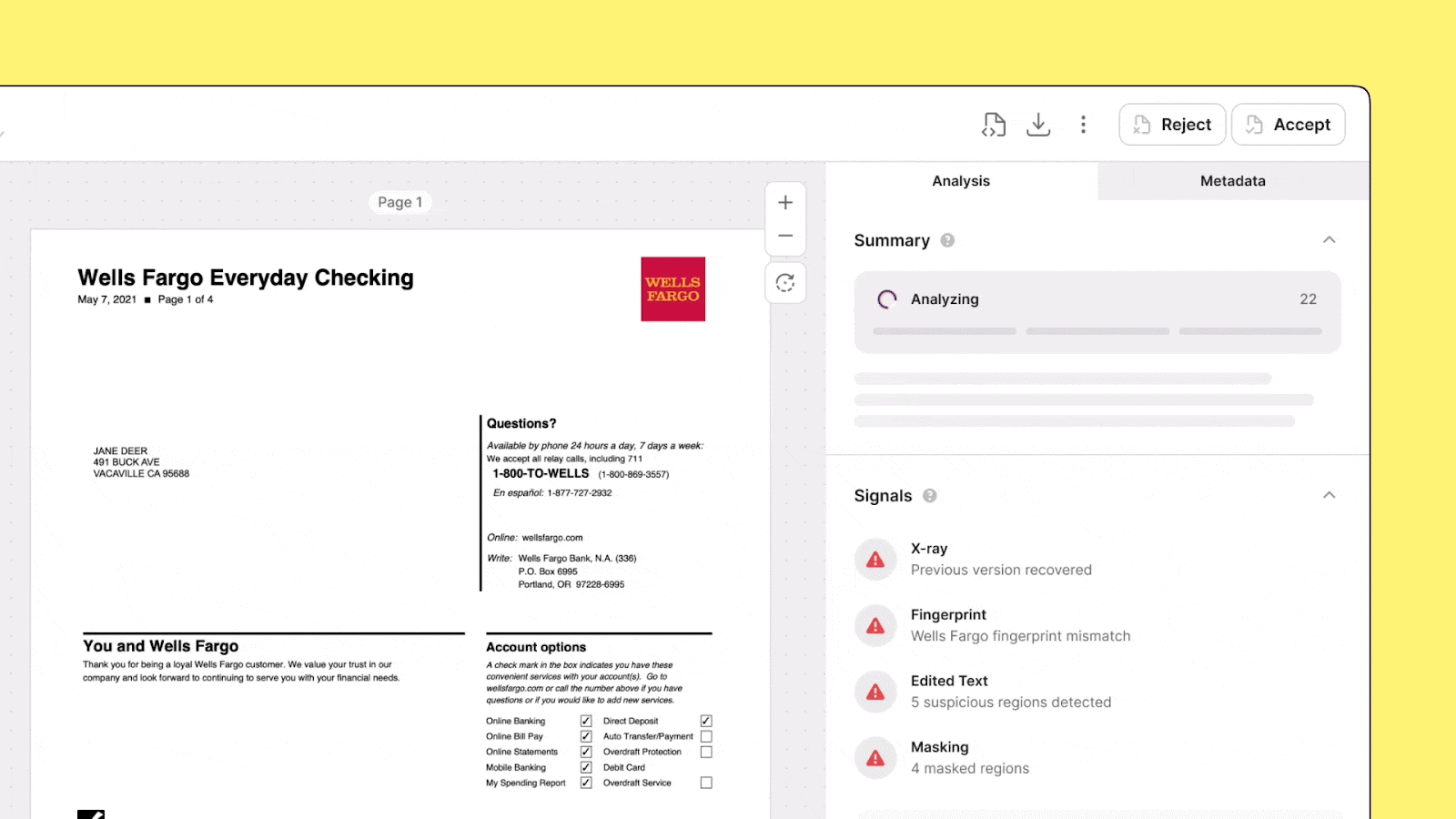
And we’re actively developing the AI Compliance Analyst, further showcasing our commitment to innovation.
As we look forward, we remain dedicated to pushing the boundaries of what’s possible with AI for risk teams.
If you’re just getting started with your AI journey or are looking to leverage the latest AI and ML advancements, Inscribe can help. Simply schedule a meeting with our team.